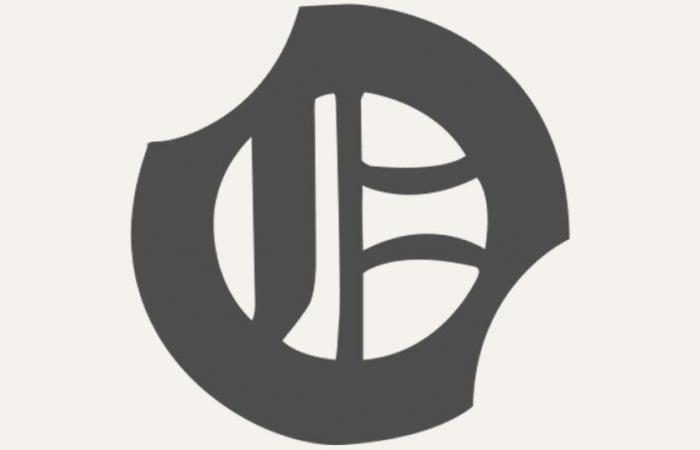
Facial recognition, automatic translation, tumor search: so many advances made possible thanks to artificial learning networks, for which John Hopfield and Geoffrey Hinton received the 2024 Nobel Prize in Physics at the beginning of October. Thanks to their pioneering work, the computer no longer simply applies a series of instructions, it
learns “by example”.
Hopfield’s associative memory
The principle of “machine learning” is inspired by the functioning of the human brain, and more particularly neural networks. In humans, learning strengthens the connections between some neurons and weakens those of others. Which, for example, draws a sort of map of connections for a given image. Physicist John Hopfield transposed this operation in 1982 to an artificial network bearing his name.
In this, the network operates “with a behavior which naturally goes towards the minimum energy”, explains Damien Querlioz, CNRS researcher specializing in information processing systems at the Center for Nanosciences and Nanotechnologies.
Hopfield compared storing a pattern in network memory to the most energy-efficient path of a marble rolling through a landscape of peaks and valleys. When the network processes a pattern close to the saved pattern, the ball’s journey will be carried out with an expenditure of energy of the same order, leading it to the same place.
“Using statistical physics techniques, he showed how a simple algorithm could store certain patterns in memory, which could be found later,” explains Francis Bach, director of the SIERRA statistical learning laboratory at the School. higher normal in Paris.
Hinton’s deep learning
Geoffrey Hinton built his work on the foundations laid by Hopfield. “He showed that we can learn efficiently when we have neural networks with several layers,” explains Francis Bach. In other words: “The more layers there are, the more complex the behavior can be, and the more complex the behavior can be, the easier it is to effectively learn a desired behavior. »
Since the 1980s, the researcher has continued to “propose new learning algorithms to learn increasingly complex behaviors,” he adds. From the end of the 1980s, researchers like the Frenchman Yann Le Cun will work “on character recognition, simpler than natural images,” he says.
Data and computing power
The discipline then experienced a relative lack of interest, until the 2010s. For their discoveries to work, computing power was needed, with much more powerful computers, and above all enormous quantities of data, which are “indispensable ingredients for neural networks,” explains Mr. Querlioz.
Machines can only learn well by ingesting as many “examples of intelligence as we want them to do”.
The Nobel committee recalls that, in his article published in 1982, Hopfield used a very simple network with “less than 500 parameters to monitor”, whereas today’s giant linguistic models contain “a trillion”.
What is it for?
The great wave of deep learning of the 2010s has “revolutionized everything that involves image processing and natural language processing,” notes Francis Bach.
Damien Querlioz cites “voice assistants, facial recognition” or image creation software like DALL-E.
But these advances go well beyond what the general public perceives. “What allows you to distinguish your children on your phone’s software also allows you to recognize a tumor,” notes Francis Bach.
It also makes it possible to analyze and classify the phenomenal quantities of data recorded in fundamental physics research institutes or to sort the images and spectra collected in star observation.
Pierre CELERIER and
Benedict KING/AFP
Facial recognition, automatic translation, tumor search: so many advances made possible thanks to artificial learning networks, for which John Hopfield and Geoffrey Hinton received the 2024 Nobel Prize in Physics at the beginning of October. Thanks to their pioneering work, the computer no longer just applies a series of instructions, he learns…
- -